How one can Examine Information When You Transfer from Google Analytics to GA4
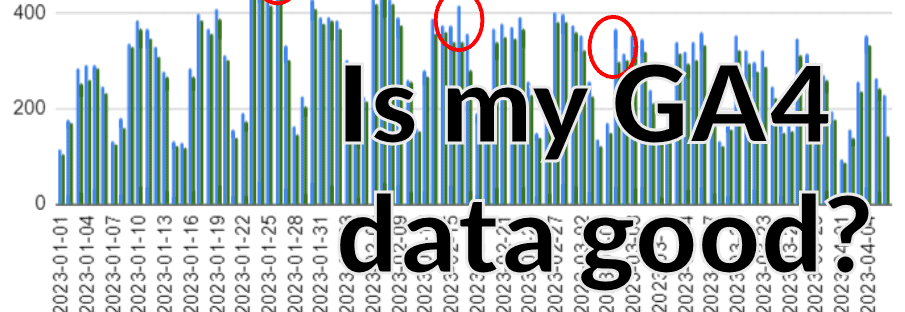
[ad_1]
How do you evaluate your knowledge whenever you transfer from Common Analytics to Google Analytics 4 (GA4), or another analytics bundle? Learn to measurement up plenty of knowledge in a short time utilizing a easy algorithm you realized in highschool.
As soon as you progress from Google Analytics to Ga4, you could be assured that your knowledge is not going to match precisely. In reality it could be off by a proportion.
What’s extra necessary is that relative modifications from each day, week to week, and month to month are of the identical magnitude in each methods.
Is the GA4 knowledge correlated with the UA knowledge?
For instance, if we graphed the Common Analytics (UA) metric Customers in opposition to the GA4 metric Lively Customers, it’d seem like this:
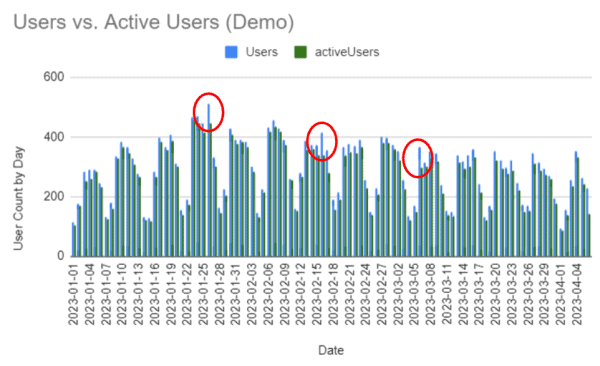
It’s clear that UA persistently stories extra Customers than GA4 lively customers on a day-by-day foundation.
The blue bars are the customers reported by UA and the inexperienced bars are the lively customers reported by GA4 every day. It’s clear that UA is reporting extra customers than GA4 is reporting acitve customers.
That is to be anticipated, as a result of lively customers are calculated in a different way by GA4 than is customers.
What’s extra necessary is that they transfer equally to one another day after day. In different phrases, if GA4 goes to report fewer lively customers, the magnitude of the distinction between it and UA ought to be constant, day after day.
For many days this seems to be true. However some days, UA reported many extra customers than GA4 reported lively customers.
Does this imply that we will’t belief one or the opposite? There’s a solution to discover out.
Scatter Plots, Not Bar Graphs
The bar graph is a crude instrument for evaluating two knowledge units. In reality, any time-series graph goes to disappoint.
What we’d like is a Scatterplot.
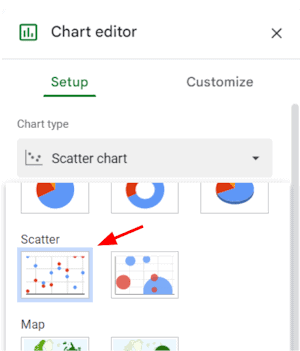
The Scatterplot graph in Google Sheets
A scatterplot ignores the order of the date and as a substitute compares the info on every day. On a day that UA reported 200 customers, what number of lively customers did GA4 report? We plot that time.
After we do it for every day in our knowledge set, we’d see one thing like this:
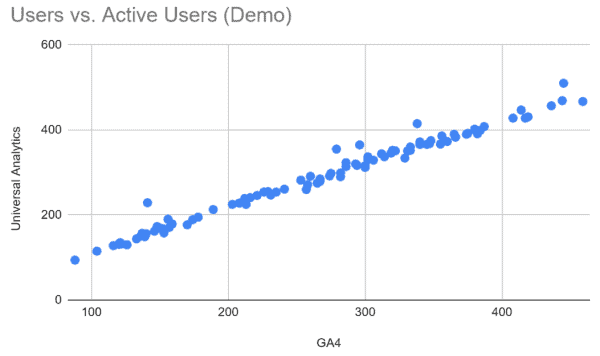
Scatterplot of UA customers vs. GA4 lively customers.
What you would possibly discover is that this knowledge lies in a straight line, for probably the most half. It is a good signal. It signifies that the GA4 knowledge modifications relative to the UA knowledge for every of the times mapped.
This doesn’t imply that it’s correct, although. Right here’s a scatterplot of the identical knowledge, however I’ve artificially doubled the each day UA knowledge.
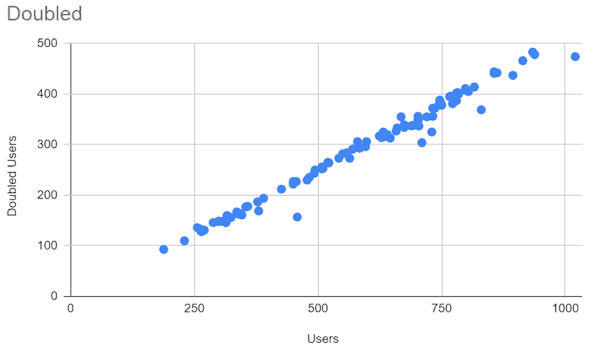
A scatterplot wherein the worth of 1 dataset has been doubled. It appears to be like just about the identical.
This knowledge appears to be like good, nevertheless it’s not. How would we all know?
Spreadsheets and your highschool math trainer give us a easy solution to consider the info like a boss.
Add a Trendline
First, Google Sheets will calculate a development line for us. When at science occasions, we name this a linear regression. That is the straight line that finest “suits” the factors. If the factors seem like a line, then the development line will probably be a detailed approximation of the info. In Google Sheets you’ll discover this within the Customise tab below Sequence >.
These options exist in Excel as nicely.
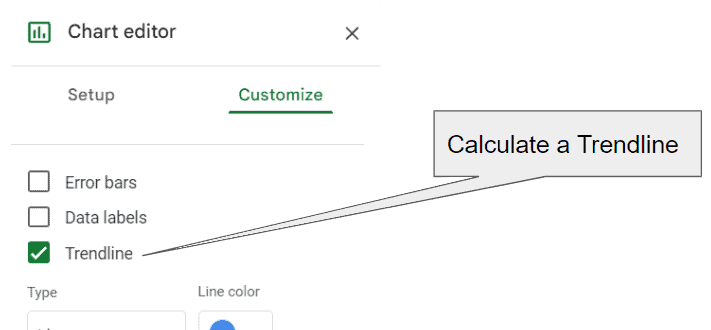
Examine the Trendline field within the Chart Editor of Google Sheets
After we add a development line to our knowledge, we see this:
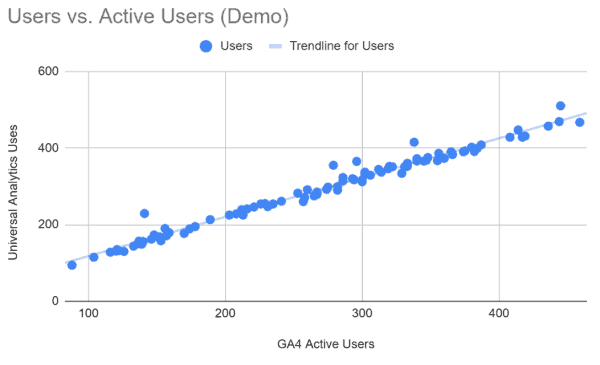
The development line matches the info very carefully. However how carefully?
That line attracts a reasonably line proper together with our knowledge. How carefully do the 2 knowledge units match? That’s what R2 tells us.
Studying the R2 Worth
If you happen to’re inquisitive about how that is calculated, right here’s a useful video.
Google Sheets will calculate R2, however this isn’t sufficient. We would like the equation of the development line in order that we all know how carefully associated the 2 knowledge units are.
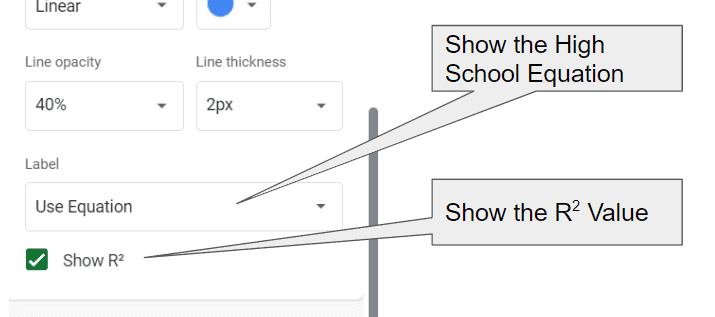
In Google Sheets, set the Label area to “Use Equation” and verify the field labeled “Present R squared”.
There are some mathy wanting bits in our legend now.
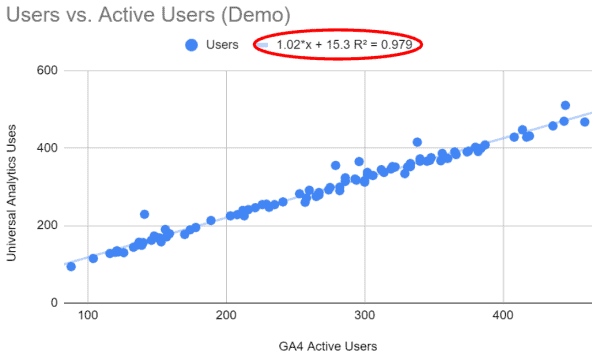
The R squared worth tells us how carefully the info “suits” our development line and the equation describes the development line intimately.
The R2 quantity tells us how nicely the development line describes our knowledge. An ideal match would give us an R2 worth of 1. The nearer to at least one it’s, the extra possible our two knowledge units are describing the identical factor.
The equation is the one you realized in highschool. It’s simply the equation of a line.
The Equation of a Development Line
That is a kind of equations that you simply swore you’d by no means use in math class. In the present day, it’s going to provide you X-ray imaginative and prescient into your knowledge.
y = mx + B
x is the GA4 Lively Customers
y is the UA Customers
The selection of x or y axis is unfair for a scatterplot.
m is the “slope” of the road. It’s the “rise over the run”. If we wish our two datasets to be alike, we wish a slope very shut to at least one.
B is the “y intercept”. It’s the place our line crosse he vertical axis, additionally known as the “y axis” when x is zero.
We’re hoping that our GA4 knowledge is as very like our UA knowledge as potential. If the 2 had been reporting the very same quantity every day:
- R2 could be 1
- The slope (m) of the road would 1
- The y intercept (B) could be 0
I in contrast two an identical knowledge units to point out this.
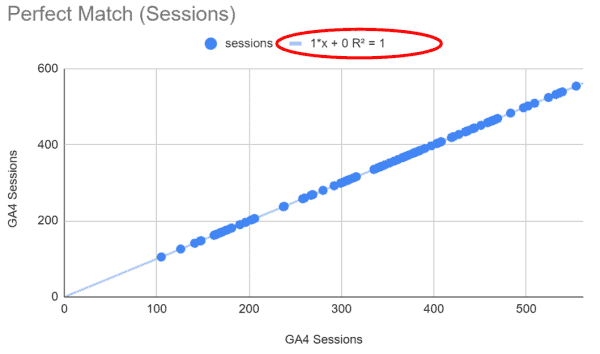
Two an identical knowledge units. The R squared worth is 1. The slope is 1. The y-intercept is 0.
So, what if our knowledge isn’t good?
If R2 is considerably lower than 0.90, then the 2 knowledge units will not be correlated to one another. In different phrases, they aren’t describing the identical factor.
Even when R2 is shut to at least one, if the slope (proper earlier than “x”) is considerably lower than one, then the 2 knowledge units are persistently improper.
Listed here are some widespread situations we see in evaluating UA and GA4 knowledge, and the way the equation could be anticipated to vary.
You’re evaluating the improper knowledge.
Let’s begin off by taking a look at a foul correlation. Right here the R2 worth and slope are close to 0. The y-intercept may be very excessive.
Somethings simply not proper right here. Possibly you’re not pulling the info proper.
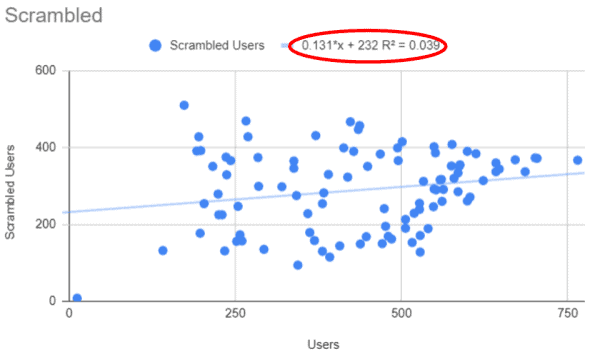
Each the R2 worth and slope are close to 0. The y-intercept is approach above 0. That is knowledge that doesn’t correlate.
Bot site visitors isn’t being filtered in a single dataset.
On this instance, I’ve added 50 customers per day to one of many datasets. That is what it might seem like if GA4 was filtering out a constant site visitors supply, like bot site visitors, however UA was not.
All the development line will is lifted by 50 customers. As a result of it’s constant, the slope and R2 values will not be affected. However the y-intercept will rise precariously.
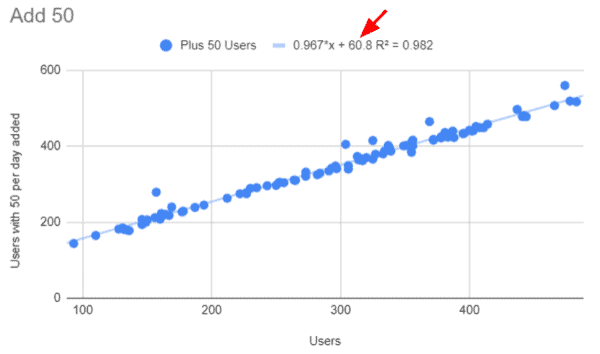
Including 50 customers to at least one dataset will increase the y-intercept, though the slope and R2 values are close to one.
You’re double counting.
It’s remarkably straightforward to double-count. The slope will probably be near 0.5 (or 2.0 in the event you flip the x and y axis in your scatterplat).
If you’re “breaking periods” in both dataset, you’ll see inflation of periods.
For instance, in the event you use a utm_ question parameter on a call-to-action button, UA will begin a brand new session, as if the person was returning to the positioning. GA4 doesn’t do that.
If you happen to’re guests are going to a third-party website and returning, you will get damaged periods. When you have cross-domain monitoring setup in UA however not in GA4, you’ll see one thing like this for the segement of tourists that go to the opposite website.
It’s commonplace for us to discover a web site that’s including pageviews utilizing an on-page tag and a tag supervisor tag. This can double-count pageviews.
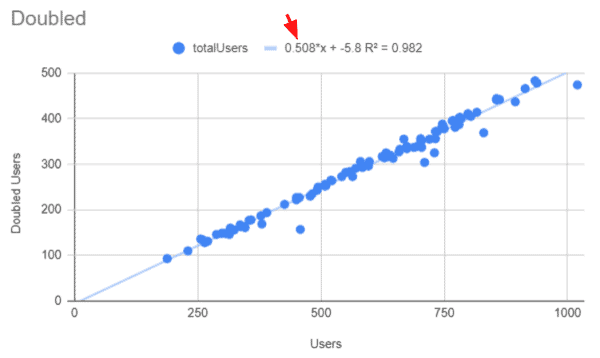
Whenever you double the customers reported in a single dataset, the slope will method 0.5 or 2.0.
The analytics tag is lacking on some pages.
With this instance, I’ve added 50% to the dataset on the Y axis. This simulates the state of affairs wherein 33% of the pages on the X-axis dataset don’t have tags.
Be aware that the R2 worth doesn’t change. Nevertheless, the slope of the road is nicely beneath 1. In reality, it’s about 2/3 of an ideal slope.
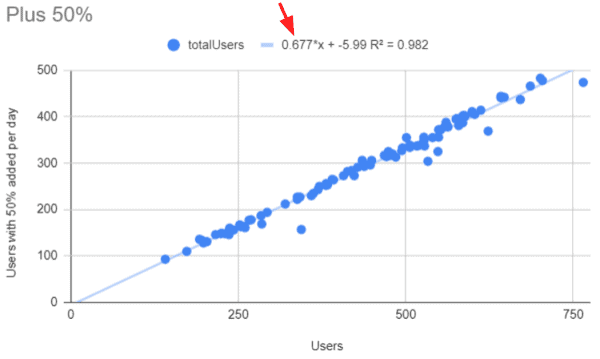
When including 50% to at least one dataset, you discover the slope altering though the R-squared worth is identical.
Income, Transacations and Segments
This method can be utilized to verify most of your metrics and segments.
Not solely are you able to consider the info you’re amassing, you may consider your skill to drag knowledge in GA4 that represents the pondering of the UA builders. UA knowledge is pre-processed in a different way in UA than it’s in GA4.
It is a smart way to make certain you’re pulling comparable knowledge segments.
You must also use this method to check in case your analytics is recording the identical transactions and income as your ecommerce backend. This is among the first issues we do with or new Conversion Catalyst shoppers.
The graphs look the identical. Don’t be fooled.
Watch out whenever you transfer from Google Analytics to GA4.
In all of those examples, the scatterplots look just about the identical visually. Nevertheless, our highschool math trainer has outfitted us with the equation we have to diagnose our knowledge.
Thanks, highschool math trainer!
[ad_2]
Source_link