Graphcore IPUs resolve visitors puzzles
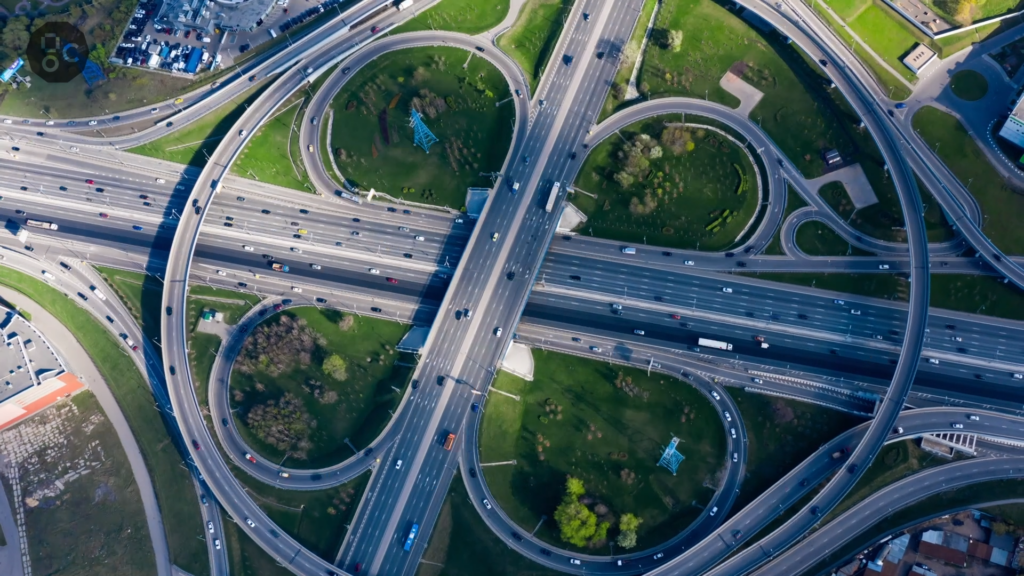
[ad_1]
Synthetic intelligence is proving to be a strong and versatile device. In monetary companies, AI can fight bank card fraud and detect market sentiment to help traders. Algorithms can generalize cyber threats to make laptop networks safer and, by the identical token, even create on-brand audio for builders of hands-free units. However there are some issues the place standard AI strategies can fall brief. And a type of use instances is journey optimization.

Supply: Unsplash
Predicting journey occasions in congested cities is a notoriously advanced activity. Builders are confronted with a shifting goal the place eventualities can change rapidly. Free-flowing visitors could all of the sudden come to a halt – for instance, if a supply van briefly blocks a street – with cascading results. AI can digest historic knowledge and establish patterns, however first responders, courier corporations, commuters, and different city-goers want real-time recommendation.
IPU enhance with GNNs
That is the place graph neural networks (GNNs) – deployed on environment friendly Intelligence Processing Models (IPUs) – come into play. A graph, on this context, is a structured illustration of information and will be pictured as a community of interconnected nodes. Right here, the nodes are particular person street sections and the hyperlinks between them (referred to as edges) signify the connection between these entities.
Graphs enable builders to assemble an in depth image of town by encoding varied data at every node. And operating this construction via a neural community aggregates the info inside the context of neighboring nodes. The method takes a fancy relationship, such because the interactions between visitors components throughout a metropolis, and prepares the data in order that it may be understood and optimized.
Working these routines on devoted IPUs – which have been designed to carry full machine studying fashions inside their processors – opens the door to extra insights. IPUs have been proven to hurry up calculations in contrast with utilizing graphics processing items (GPUs), and are significantly environment friendly at accelerating GNNs regardless of their advanced graph buildings. This speed-up is very worthwhile when the top purpose is to carry out journey optimization in real-time.
Well timed recommendation
Another excuse for utilizing IPUs on clever transportation methods is the capability to carry out so-called Combination of Specialists (MoE) visitors mannequin integration. Moderately than counting on a single international view, totally different behaviors will be fed into the pipeline – for instance, rush hour circumstances or weekend patterns. The benefit is an uplift in prediction accuracy. However with GPUs, the computational burden of getting to accommodate a number of fashions would decelerate the system. Not so for IPUs.
“[The IPU] permits a number of directions and a number of knowledge to be processed on totally different tiles,” feedback Chen-Khong Tham – a professor primarily based on the Nationwide College of Singapore, who has used MoE mannequin integration to enhance visitors forecasting. “That is very helpful when you’ve got operations that aren’t homogeneous.”
Recognizing that visitors patterns change over time, Tham and his colleagues have been utilizing a sandwiched workflow that takes each spatial and temporal options into consideration. Within the last step, AI is deployed to foretell future visitors speeds on totally different street sections to help route planning and journey optimization. Because of the usage of IPUs – developed by UK agency Graphcore – the staff noticed a speedup of as much as 4X (in contrast with GPUs) in processing knowledge from over 39,000 sensors distributed throughout main metropolitan areas of the California state freeway.
Releasing up metropolis visitors via journey optimization – enabled by graph neural networks operating on IPUs – may have important financial advantages. On-line supply corporations, an trade that has accelerated in recent times, may enhance their fleet administration capabilities. Fashions may reply to new elements too such because the emergence of fresh air zones and different metropolis planning initiatives.

Supply: Unsplash
GNN traits
Graph knowledge buildings and neural networks are turning out to be a sensible mixture throughout a spread of purposes. Graphs are a helpful manner of including construction to AI fashions in order that they will carry out effectively and collect regionally related options – for instance, replicating the success of picture recognition algorithms. In contrast with utilizing AI alone, the addition of graphs can assist to mannequin new methods, present knowledge effectivity, and end in quicker coaching.
Combining these advantages with the made-to-measure match of IPUs which have been designed with massively parallel processing in thoughts, provides builders a really highly effective analytical mixture certainly. And it’s why GNNs operating on IPUs are being utilized in varied sectors not simply in transport and the “sensible metropolis.” Different software areas embody molecular evaluation, drug discovery, inventory market prediction, social community evaluation, and recommender methods for e-commerce, to provide just some examples.
To learn the way IPUs can improve your AI workflow and supply a notable acceleration to processing, go to https://www.graphcore.ai.
[ad_2]
Source_link