Newest Machine Studying Analysis From Deepmind Explores The Connection Between Gradient-Primarily based Meta-Studying And Convex Optimization
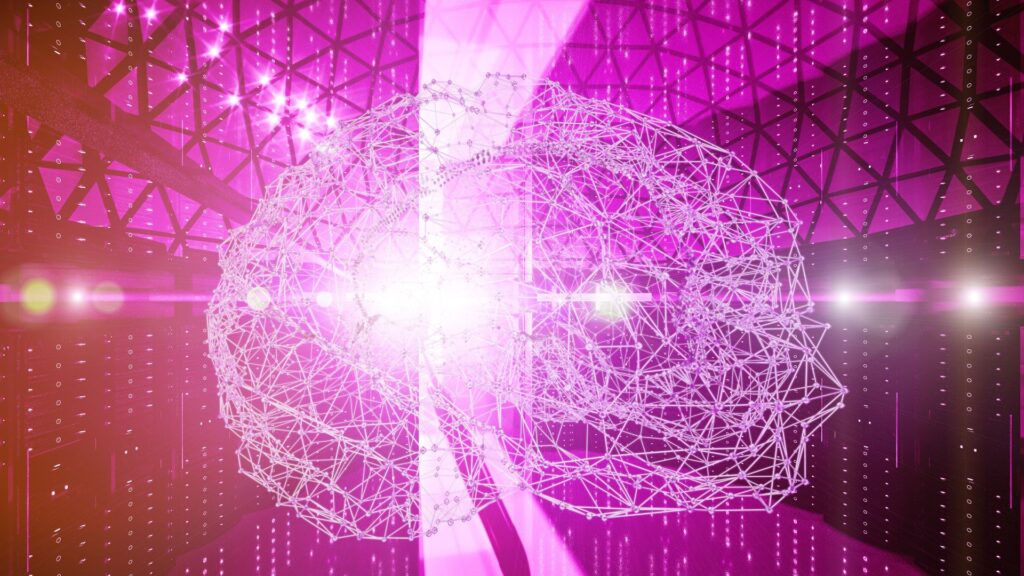
[ad_1]
The time period “meta-learning” refers back to the course of by which a learner adjusts to a brand new problem by modifying an algorithm with identified parameters. The algorithm’s parameters are meta-learned by measuring the learner’s progress and adjusting accordingly. There’s lots of empirical help for this framework. It has been utilized in varied contexts, together with meta-learning, the right way to discover reinforcement studying (RL), the invention of black-box loss features, algorithms, and even full coaching protocols.
Even so, nothing is known in regards to the theoretical options of meta-learning. The intricate relationship between the learner and the meta-learner is the primary motive behind this. The learner’s problem is optimizing the parameters of a stochastic goal to reduce the anticipated loss.
Optimism (a forecast of the long run gradient) in meta-learning is feasible utilizing the Bootstrapped Meta-Gradients method, as explored by a DeepMind analysis group of their latest publication Optimistic Meta-Gradients.
Most earlier analysis has centered on meta-optimization as a web based drawback, and convergence ensures have been derived from that perspective. Not like different works, this one views meta-learning as a non-linear change to conventional optimization. As such, a meta-learner ought to tune its meta-parameters for optimum replace effectivity.
The researchers first analyze meta-learning with trendy convex optimization methods, throughout which they validate the elevated charges of convergence and take into account the optimism related to meta-learning within the convex scenario. After that, they current the primary proof of convergence for the BMG method and show the way it could also be used to speak optimism in meta-learning.
By contrasting momentum with meta-learned step dimension, the group discovers that incorporating a non-linearity replace algorithm can improve the convergence price. With the intention to confirm that meta-learning the size vector reliably accelerates convergence, the group additionally compares it to an AdaGrad sub-gradient method for stochastic optimization. Lastly, the group contrasts optimistic meta-learning with conventional meta-learning with out optimism and finds that the latter is considerably extra prone to result in acceleration.
Total, this work verifies optimism’s perform in rushing up meta-learning and presents new insights into the connection between convex optimization and meta-learning. The outcomes of this examine suggest that introducing hope into the meta-learning course of is essential if acceleration is to be realized. When the meta-learner is given cues, optimism comes naturally from a classical optimization perspective. A serious enhance in velocity might be achieved if clues precisely predict the training dynamics. Their findings give the primary rigorous proof of convergence for BMG and a normal situation below which optimism in BMG delivers fast studying as targets in BMG and clues in optimistic on-line studying commute.
Try the Paper. All Credit score For This Analysis Goes To the Researchers on This Challenge. Additionally, don’t neglect to affix our Reddit Web page, Discord Channel, and E-mail Publication, the place we share the most recent AI analysis information, cool AI initiatives, and extra.
Tanushree Shenwai is a consulting intern at MarktechPost. She is at the moment pursuing her B.Tech from the Indian Institute of Know-how(IIT), Bhubaneswar. She is a Information Science fanatic and has a eager curiosity within the scope of software of synthetic intelligence in varied fields. She is keen about exploring the brand new developments in applied sciences and their real-life software.
[ad_2]
Source_link