What Is Statistical Energy And How Do You Measure It

[ad_1]
Should you’re concerned in digital advertising and marketing or product growth not directly, you already know simply how important A/B testing is for making data-driven selections.
On the coronary heart of A/B testing is statistical energy. That is truly a crucial element in figuring out the take a look at’s effectiveness in detecting variations between variants.
This text discusses statistical energy in A/B testing intimately, supplying you with full insights into what it’s, the components affecting it, and the steps concerned in measuring it.
From pre-test evaluation, together with estimating pattern measurement and figuring out impact measurement, to post-test evaluation, deciphering outcomes, and making knowledgeable selections, this text covers every section of A/B testing with detailed insights and sensible approaches.
What Is Statistical Energy in A/B Testing?
Statistical energy in A/B testing is an important idea. It determines the effectiveness of the take a look at in detecting an precise impact if there may be one.
In easier phrases, it’s the take a look at’s capability to detect a change or enchancment when one model is healthier or completely different.
What makes statistical energy so essential in A/B testing?
Excessive statistical energy means a better likelihood of detecting a real constructive (an actual distinction between teams A and B). Low energy will increase the chance of a Sort II error (failing to detect an actual impact), resulting in doubtlessly incorrect conclusions in regards to the effectiveness of the variables being examined.
Listed below are some components which may affect the statistical energy:
- Impact Measurement: Bigger impact sizes (variations between teams) are simpler to detect, rising energy.
- Pattern Measurement: Bigger pattern sizes typically enhance energy, extra precisely representing the examined populations.
- Significance Stage (Alpha): Setting a decrease significance degree (e.g., 0.01 as a substitute of 0.05) makes the take a look at extra stringent however can lower energy.
- Variability in Knowledge: Much less variability inside every group results in increased energy, as detecting a distinction between teams is simpler.
Measuring Statistical Energy in A/B Testing
Pre-Check Evaluation
1. Estimating Required Pattern Measurement
The pattern measurement is the variety of observations or knowledge factors (corresponding to customers or transactions) wanted in every group of the A/B take a look at to detect a distinction if one exists reliably.
In case your pattern measurement is simply too small, it might result in unreliable outcomes. Alternatively, in case your pattern measurement is simply too massive, it might waste sources.
Earlier than calculating the pattern measurement, you have to outline the anticipated impact measurement – the distinction you count on to see between the 2 teams. You may base this on business requirements, earlier checks, or enterprise targets.
The following step is to pick out a Significance Stage (Alpha). Sometimes set at 0.05, that is the likelihood of rejecting the null speculation when it’s true (Sort I error). A decrease alpha reduces the possibility of false positives however requires a bigger pattern measurement.’
After that, select a Energy Stage (1 – Beta). Generally set at 0.80 or 0.90, that is the likelihood of accurately rejecting the null speculation when the choice speculation is true. Increased energy requires a bigger pattern measurement.
Lastly, estimate the variability or customary deviation in your knowledge. Extra variability means you’ll want a bigger pattern measurement to detect an impact.
To conduct the ultimate calculations, you should use statistical formulation incorporating the impact measurement, significance degree, desired energy, and variability.
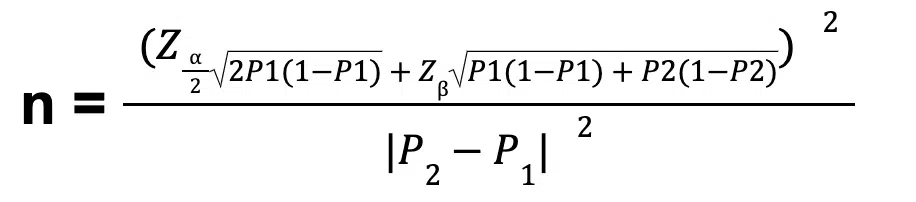
Statistical method (Supply)
From the method above, listed below are all of the variables acknowledged:
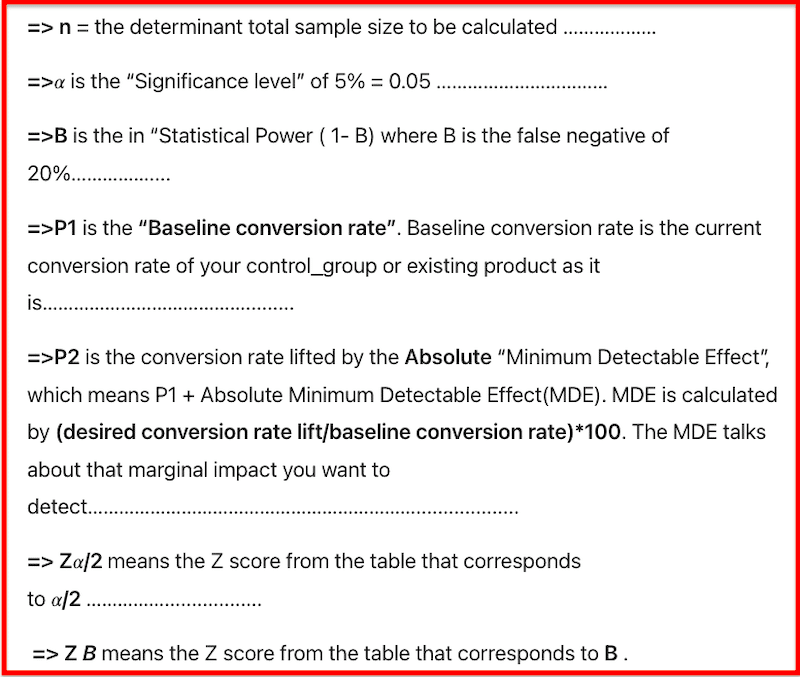
Statistical method variables (Supply)
Alternatively, you should use numerous on-line calculators and statistical software program instruments out there to simplify this calculation of pattern sizes by inputting the specified impact measurement, alpha, energy, and variability.
2. Figuring out Impact Measurement
Impact measurement is the magnitude of the distinction between teams that the take a look at is designed to detect. It’s a measure of how impactful the change is.
Understanding the impact measurement helps in understanding the sensible significance of the take a look at outcomes, thereby serving to you calculate the pattern measurement and set lifelike expectations.
Listed below are some methods that can assist you decide your impact measurement:
- Imply distinction: A standard option to calculate the impact measurement for steady knowledge is Cohen’s d, which is the distinction between two means divided by the usual deviation.
- Proportional distinction for charges and proportions: For binary outcomes (like conversion fee), the impact measurement may be the distinction in proportions or a ratio.
- Utilizing pilot research: Conducting a small-scale pilot take a look at may also present preliminary knowledge to estimate a extra correct impact measurement.
3. Selecting an Acceptable Significance Stage
The importance degree, typically denoted as alpha (α), determines the edge for statistical significance and performs a significant function in deciphering take a look at outcomes.
Merely put, the importance degree is the likelihood of rejecting the null speculation when it’s truly true. In different phrases, it’s the chance of a Sort I error – falsely figuring out an impact with out one.
The commonest significance ranges in A/B testing are 0.05 (5%) and 0.01 (1%). A 5% degree means there’s a 5% likelihood of concluding {that a} distinction exists when there isn’t any precise distinction.
Typically, the tester chooses the alpha degree earlier than the take a look at begins, and it’s not adjusted based mostly on the info. This mounted method prevents bias within the interpretation of outcomes.
Conducting the A/B Check
Now that you simply’ve performed the pre-test evaluation, it’s lastly time to conduct the A/B take a look at. Right here’s find out how to go in regards to the course of:
1. Implementing the Check Design
This section entails placing into motion the plan developed in the course of the pre-test evaluation – ensuring you execute the take a look at in a means that yields dependable and legit outcomes.
Earlier than you begin, affirm the weather you intend to check (e.g., webpage design, e-mail marketing campaign content material). Guarantee these parts are well-defined and the adjustments between the management (A) and variant (B) are clear and measurable.
After that, decide find out how to choose and assign the individuals or knowledge factors to the management or take a look at group. That is sometimes performed by means of randomization to make sure that the 2 teams are comparable and that the outcomes usually are not biased by exterior components.
For the uninitiated, randomization helps in controlling for confounding variables and ensures that any noticed impact may be attributed to the adjustments being examined.
After that, observe this step-by-step course of to implement the take a look at design:
- Pattern Measurement and Energy Concerns: Implement the pattern measurement determined upon within the pre-test evaluation. Do not forget that the pattern measurement should meet the necessities to realize the specified statistical energy.
- Setting Up the Check Atmosphere: Be certain that the testing surroundings is managed and constant. For on-line testing, this would possibly contain organising the mandatory software program instruments or platforms to show the variations to completely different customers.
- Monitoring for Check Integrity: All through the take a look at, monitor for any points which may have an effect on its integrity, corresponding to technical issues or surprising exterior components.
- Knowledge Assortment: Accumulate knowledge rigorously and make sure you acquire all of the related data. This contains not solely the first metric(s) of curiosity but additionally any auxiliary knowledge which may assist in deciphering the outcomes (extra on this within the subsequent part).
Whereas implementing the take a look at design, be sure you keep away from sources of bias, together with choice bias, measurement bias, or experimenter bias. Be certain that the strategies of information assortment and evaluation are goal and constant.
2. Knowledge Assortment Strategies
After you implement the take a look at design, the following step is to gather the info meticulously to make sure the reliability and accuracy of the outcomes.
The easiest way to go about it’s by utilizing software program instruments and applied sciences suited to A/B testing. For web sites or apps, this will likely embody analytics platforms like FigPii or custom-built instruments.
Listed below are the info assortment strategies you should use:
- Random Sampling: Randomly assign individuals or knowledge factors to both the management group or the experimental group to keep away from choice bias and be certain that the teams are comparable.
- Person Monitoring: Don’t neglect to trace consumer interactions with the product by means of cookies, session IDs, and extra.
- Time-Stamping: Document the time when knowledge is collected. This may assist you to decide how outcomes might fluctuate over completely different intervals and controlling for time-based variables.
- Automated Knowledge Assortment: Automation helps in gathering massive volumes of information with minimal error. This may embody automated occasion monitoring on web sites or apps.
- Qualitative Knowledge Assortment: Along with quantitative knowledge, qualitative knowledge, like consumer suggestions, will provide you with insights into sure consumer behaviors or preferences.
Publish-Check Evaluation
1. Calculating Noticed Energy
The noticed energy is the likelihood that the take a look at accurately rejected the null speculation (which states that there isn’t any distinction between the 2 teams) given the noticed impact measurement, pattern measurement, and significance degree.
It’s a post-hoc evaluation that tells you the way possible your take a look at was to detect the impact you noticed.
Calculating noticed energy helps in deciphering the outcomes of the A/B take a look at. If a take a look at has low noticed energy, any non-significant findings (failure to reject the null speculation) may be because of the take a look at not being delicate sufficient somewhat than the absence of an actual impact.
So, how will you take a look at the noticed energy? Listed below are some ideas:
- Utilizing Statistical Software program: You should utilize statistical software program like R, Python, or specialised instruments like G*Energy to calculate the noticed energy. These instruments have built-in capabilities to calculate energy based mostly on the take a look at’s parameters.
- Enter Parameters: To calculate noticed energy, you want the noticed impact measurement out of your take a look at, the pattern measurement of every group, and the alpha degree (significance degree) you used.
- Statistical Strategies: The calculation typically entails figuring out the non-centrality parameter based mostly on the noticed impact measurement after which utilizing this parameter to seek out the facility. For instance, in a two-sample t-test, the non-centrality parameter is calculated, after which the cumulative distribution perform is used to seek out the facility.
2. Decoding the Outcomes
This course of entails wanting past the mere statistical significance to know the sensible implications of the outcomes.
Start by figuring out if the outcomes are statistically vital. You are able to do it by evaluating the p-value out of your statistical take a look at to the pre-set alpha degree (e.g., 0.05). A p-value decrease than the alpha degree signifies statistical significance.
That mentioned, statistical significance alone received’t provide you with an thought of sensible significance.
Ensure you consider the impact measurement, which refers back to the distinction between the 2 teams. For instance, a small however statistically vital impact won’t be virtually related.
And as we’ve already mentioned, evaluate the noticed energy of the take a look at. A excessive noticed energy strengthens confidence within the outcomes, particularly if the take a look at outcomes usually are not vital.
3. Making Selections Based mostly on Check Outcomes
When you achieve insights into the take a look at, it’s time to show these insights into actionable steps.
This course of entails deciphering the take a look at ends in the context of enterprise or analysis targets after which making knowledgeable selections in regards to the implementation of adjustments based mostly on these outcomes.
Earlier than making selections, totally interpret the take a look at outcomes, contemplating statistical significance, impact measurement, and noticed energy. Perceive not simply whether or not the outcomes are statistically vital but additionally if they’re virtually vital in your particular context.
One other necessary issue is assessing how the outcomes align with enterprise objectives or analysis targets. As an example, even a slight enhance in conversion fee may be vital for a big ecommerce enterprise.
Contemplate the next when making selections based mostly in your take a look at outcomes:
- Constructive Outcomes: If the take a look at exhibits a constructive consequence (for instance, the variant performs considerably higher than the management), resolve if the development is value the price and energy of implementation. Contemplate scalability and long-term implications.
- Unfavorable or Impartial Outcomes: For detrimental or non-significant outcomes, decide whether or not it’s greatest to desert the adjustments, make modifications, or conduct additional testing. Generally, non-significant outcomes can present worthwhile insights for future checks.
- Danger Evaluation: Assess the dangers of implementing the adjustments based mostly on the take a look at outcomes. This contains contemplating the potential impression on consumer expertise, model notion, and potential expenditures.
Based mostly on the above outcomes, plan for a full-scale rollout in case you lastly resolve to implement the adjustments. Ensure to tell all stakeholders and make obligatory changes for a easy transition.
If the outcomes stay unsure, don’t hesitate to conduct additional checks or iterations to assemble extra knowledge and insights.
Whatever the choice, doc the take a look at course of, outcomes, and your selections. This may assist you to discuss with the doc sooner or later or construct a data base for future assessments.
Over to You!
Making sense of statistical energy is vital to profitable A/B testing. It’s like ensuring you’ve gotten a powerful sufficient magnifying glass to see the small print of your experiment clearly.
Every step is necessary, from deciding how many individuals to incorporate in your take a look at (pattern measurement) to determining what measurement of change you’re on the lookout for (impact measurement). After working the take a look at, you possibly can’t overlook figuring out whether or not your take a look at was adequate to seek out actual variations.
Lastly, you fastidiously take a look at what the numbers are telling you and resolve what to do subsequent.
In easy phrases, this information helps you be sure that your A/B checks are arrange proper, run easily, and the outcomes are utilized in the easiest way to enhance your product or technique.
[ad_2]
Source_link